For Ashley Zappia (pictured lower right), getting her hands dirty was part of her job. Even though she always tried to remain as clean as possible, her work as a nursing aide at a Southern California hospital required a lot of diapering, changing, and other hands-on tasks. She was mostly in the ER, where physical contact with bodily fluids from sick patients was normal. She was careful to wash her hands frequently, even though she almost always wore gowns and gloves with all patients. Every time she left or entered a room, she lathered her hands in hand sanitizer gel. Just 27 years old, she was young and healthy. On paper, she was at low risk for infection. That’s why, at first, she thought little of a urinary tract infection (UTI) that seemed to appear out of nowhere.
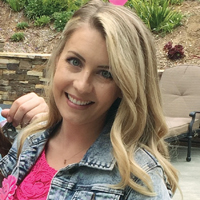
After a few days, her UTI got progressively worse, eventually landing her in the ER. Zappia received a catheter flush and a strong, five-day regimen of Cipro, a broad-spectrum antibiotic. She was then sent home. After two weeks, her UTI morphed into something wholly different—unrelenting diarrhea, fever, nausea, and extreme stomach pains. She couldn’t keep any food down. Three trips to urgent care yielded the same diagnosis—just a bad flu, they said. It would run its course. Eventually, she was hospitalized.
“I felt like I was dying,” Zappia recalls. Her heart rate and blood pressure skyrocketed. Doctors thought she was having a heart attack and put her under cardiac observation. Her diarrhea, which subsided slightly with an empty stomach, eventually returned. Fecal tests finally revealed the true source of her woes—Clostridium difficile, a bacterium commonly known as C. diff.
An Uncommon Test for a Common Condition
Most patients treated in the early stages are able to overcome an infection with relative ease, but Zappia was not one of them. After numerous failed treatments, her infection didn’t go away. Worse, the treatments seemed to make her more susceptible to other illnesses—she caught every cold and flu she was exposed to. Eventually, other blood work revealed some- thing unexpected: Zappia had selective immunoglobulin-A deficiency, a genetic immunodeficiency that both predisposed her to infection and kicked into overdrive once she was infected. Such immunodeficiencies are probably more common than is widely known but can be detected only with an uncommon test. This was likely the reason Zappia kept getting ill. Patients having this and other types of immunodeficiencies are often unaware of their condition, entering hospitals as moving targets for infection, perfect vectors for infectious disease.
C. diff is ubiquitous in the environment. A common resident of soil, water, and both human and animal feces as well as food products like processed meats, it may also be found in small quantities in a healthy human gut. Yet, as one of the most common hospital-acquired infections, C. diff has become the bane of medical facilities across the country. Infections cause inflammation of the colon and diarrhea that can easily become life-threatening. In a hospital environment, where Zappia was likely infected, infections spread quickly and are both hard to control and difficult to treat.
In 2015, the U.S. Centers for Disease Control estimated nearly half a million C. diff infections in the United States, leading to 15,000 deaths—the vast majority, roughly 80%, occurring in adults age 65 or older. A patient identified as having C. diff is quickly isolated, and healthcare workers take extra precautions like wearing gowns and gloves and using specialized cleaning products to decontaminate the local hospital environment. But these extra precautions are only taken when a diagnosis has been made.’
Identifying Risk Likelihood
The trouble is that detection is tricky. Clinicians are desperate for new ways to identify at-risk and infected patients. “I think every time I talk about this problem, someone from the audience comes up to me and tells me about a personal story, a family member who had C. diff and either is still dealing with it or has passed away,” says Erica Shenoy (left), an infectious-disease specialist at Massachusetts General Hospital. “So it’s a really nasty disease that can be treat- ed, but it will come back very often.” It’s difficult to get rid of the disease if it’s not addressed immediately, and roughly 20% of cases relapse within 60 days.

To acquire C. diff, a patient must be both susceptible and exposed to C. diff. “I could ingest C. diff and not get an infection, and someone else could ingest it and have a very severe infection,” explains Shenoy. The risk factors, she continues, may include whether a patient, like Zappia, is immunocompromised or whether his or her intestinal microbiome has been perturbed by antibiotic exposure. Patients also have to consume bacterial spores orally, which can happen easily with physical contact.
Shenoy describes a common scenario. “The healthcare worker is in a room with a patient, whether they knew or did not know they had C. diff, and attracts some of the spores on their hand,” she said. “The hands are not effectively cleaned, they go to the next patient, they shake the patient’s hand, that patient then eats their lunch and takes some C. diff spores down with their lunch.” Risk is heightened by the mere fact that the patient is in a hospital environment, where it’s common for many people to have C. diff, recognized or unrecognized, Shenoy suggests.
But, now, data that used to be on paper charts, and maybe left unseen in a storage facility for years on end, are available in electronic health records (EHRs). This has opened a world of new possibilities for tackling hospital infections.
Devils in the Data
The complex nature of C. diff infection and the widespread use of EHRs are irresistible to software engineers like Jenna Wiens (lower right), a professor of computer science and engineering at the University of Michigan in Ann Arbor. Her lab, which focuses on developing machine-learning techniques for health, is working with Shenoy on a new way to detect and prevent hospital C. diff infections using artificial intelligence (AI). It’s an approach made possible by the massive quantities of digital patient data acquired by hospitals with EHRs; much of these data were never readily available on paper. In most EHRs, everything is available in one place—the amount of time a patient spends in a particular space, the people with whom he or she spend it, and more.

“We’re collecting just an immense amount of patient data, everything from medications to lab results to where patients are in the hospital,” says Wiens. “We should be using the data to improve patient care.” But, although clinicians are spending more time with patient data, they still end up ignoring the vast majority, she explains. Wiens believes the answers to unlocking and making the data more usable lie in the data matrix, among what were once previously indecipherable patterns now visible through machine learning.
Machine learning is modeled after the animal brain. Just like our own neural networks, a computer can be trained to sort variables into distinct patterns that can be predictive of an outcome—risk, reward, danger. A scraped bowl of batter next to a hot oven, accompanied by the smell of cinnamon and butter might, for example, make your mouth water in anticipation of some scrumptious cinnamon rolls. Similarly, an immunocompromised patient like Zappia, who worked in a busy ER with a high rate of C. diff infection and was often exposed to bodily fluids, would have fit into a pattern of increased risk for infection. A computer keeps track of these details, many more than healthcare professionals might be capable of processing on their own. It just needs to be trained to find meaning in that sea of information.
Wiens initially acquired data from 200,000 patients, teasing out the individual risk factors for a C. diff infection to which each patient was exposed. From that data, she found patterns to predict an individual patient’s probability of acquiring an infection during a stay at the hospital. After identifying high-risk patients, her algorithm may also recommend the best intervention, depending on a patient’s circumstances.
“Ideally, we would be able to predict who is at risk before they get C. diff,” explains Shenoy, making treatment unnecessary for those not at risk. “As an individual physician, diligent as I am with my patients, I cannot conceive of all of these risk factors that are out there,” she continues. “I think this technology is the logical next step.”
A New Era of Intelligence
AI is poised to transform health care. It is already being used to diagnose various forms of cancer, and, in 2016, the Cleveland Clinic announced a collaboration with Microsoft that aims to identify intensive care unit patients at risk for cardiac arrest using AI. Massachusetts General Hospital, Shenoy’s home base, established the hospital’s Clinical Data Science Center, with the goal of making it a key player in AI applications in health care. And these changes are occurring beyond the United States as well. The Guangzhou Second Provincial Central Hospital in China has incorporated AI into almost all of its hospital operations—prediagnosis, CT scans, organizing patient records, and transporting operating-room supplies. It is even used as a diagnostic tool, working off a database containing over 300 million medical records.
For their part, Wiens and Shenoy have already shown their algorithm to be effective in hospitals in the District of Columbia, Boston, Chicago, and Michigan. They’ve also made their code available in the public domain, accessible to any hospital or clinic with electronic records. All that’s needed is personnel with enough experience to run the code. Operational costs, therefore, are minimal.
Some argue that AI for health care is a disruptive technology, poised to steal jobs from healthcare professionals. They fear machines will eventually replace even doctors and nurses, a scenario already playing out at the University College of London Hospitals, where plans are being made to use AI to perform sophisticated diagnostic tasks and identify patients most at risk for infections like C. diff.
“That’s definitely not the goal of this project or really any project in machine learning,” Wiens says. “The goal is not to replace clinicians but augment clinicians—provide them with additional information that they can then take and apply to improve patient care.” The real challenge, Shenoy argues, will be to identify the best audience for the information they generate. Alert fatigue, where a rapid influx of information is eventually ignored by the recipient, could become an issue if records are delivered to the wrong recipient. “A better audience for C. diff risk factors might be the infection-control group within an institution or the antimicrobial stewardship program,” as opposed to a clinician, Shenoy explains. But the idea was never to entirely replace a human with a machine.
The Road Ahead
Nearly three years after her initial diagnosis, Zappia is still coping with her C. diff. She is no longer working directly with patients, serving instead as a financial advocate, helping patients by phone to access financial services to pay for their health services. She has undergone two fecal microbiota transplants to restore bacterial populations in her gut. The first failed, and the second seemed to be working until her symptoms reappeared earlier this year. Stool samples indicated she was still positive. “My soul was crushed,” she said. “I had to go see a therapist for what they described as posttraumatic stress disorder from an infection, which is weird to say but it’s true. I was afraid to eat. I was afraid to do everything.”
Currently, her symptoms seem to have subsided, but food allergies have made her intolerant of dairy, uncooked vegetables, onions, and garlic. Most of her doctors are at a loss as to what to do. It is probably unlikely she will ever completely rid herself of C. diff, something she probably could have done had the infection been identified early on. “It’s easy to fall through the cracks and not be properly treated,” she continues. “It would be nice if we had better procedure and protocol.”
Works Consulted
- J. Wiens and E. S. Shenoy, “Machine learning for healthcare: On the verge of a major shift in healthcare epidemiology,” Clinical Infectious Diseases, vol. 66, no. 1, pp. 149–153, 2017.
- CDC Newsroom. (2015, Feb. 25). Nearly half a million Americans suffered from Clostridium difficile infections in a single year. Centers for Disease Control and Prevention. Atlanta, GA. [Online].
- S. Gauher and F. Uz. (2016, Sept. 26). Cleveland Clinic to identify at-risk patients in ICU using Cortana Intelligence. Microsoft Corp. Redmond, WA. [Online].
- NVIDIA. (2016, Apr. 5). NVIDIA, Massachusetts General Hospital use artificial intelligence to advance radiology, pathology, genomics. NVIDIA. Santa Clara, CA. [Online].