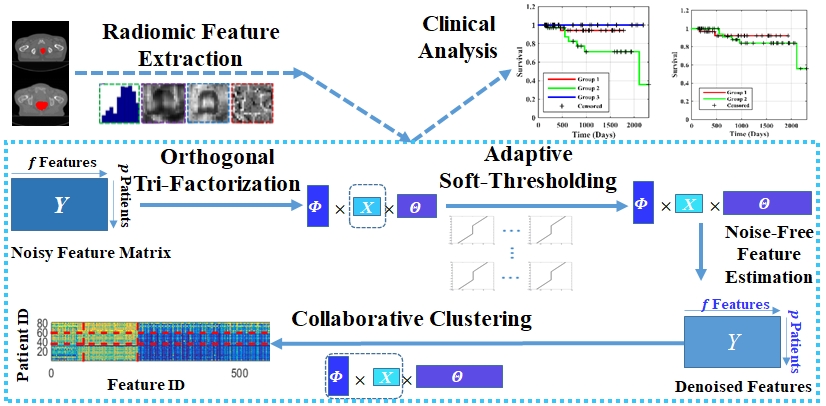
Feature dimensionality reduction plays an important role in radiomic studies with a large number of imaging features. However, conventional radiomic approaches may suffer from noise, and most existing feature dimensionality reduction techniques are not equipped to utilize latent supervision information of patient data under study, such as differences in patients, to learn discriminative low dimensional representations.
To achieve robustness to noise and feature dimensionality reduction with improved discriminative power, we develop a robust collaborative clustering method to simultaneously cluster patients and radiomic features into distinct groups respectively under adaptive sparse regularization. Our method is built upon matrix tri-factorization enhanced by adaptive sparsity regularization for simultaneous feature dimensionality reduction and denoising. Particularly, latent grouping information of patients with distinct radiomic features is learned and utilized as supervision information to guide the feature dimensionality reduction, and noise in radiomic features is adaptively isolated in a Bayesian framework under a general assumption of Laplacian distributions of transform-domain coefficients.
The method has been evaluated on both synthetic and real datasets. Experiment results on synthetic data have demonstrated the effectiveness of the proposed approach in data clustering, and evaluation results on an FDG-PET/CT dataset of rectal cancer patients have demonstrated that the proposed method outperforms alternative methods in terms of both patient stratification and prediction of patient clinical outcomes. These experiment results suggest that integrating feature denoising and unsupervised collaborative clustering into a robust system could improve cancer patient stratification and prognosis.